Imagine cutting your financial analysis time from hours to minutes—how would that impact your competitive edge? Automating financial spreading is transforming risk assessment and credit analysis, drastically reducing the labor-intensive process traditionally relying on manual data entry and complex spreadsheets.
Leveraging machine learning algorithms, these tools quickly extract, categorize, and analyze financial data from diverse sources, automating the spreading process to improve productivity and enhance the consistency and quality of financial analysis, minimizing errors and strengthening the accuracy of risk assessments, providing financial institutions with a more reliable foundation for strategic decision-making.
Financial landscapes evolve rapidly, making real-time data processing essential. Automated financial spreading tools ensure compliance with regulatory standards, allowing institutions to react promptly to market changes and make well-informed decisions in a fast-paced environment. By integrating these systems, financial institutions gain an agile edge, maintaining business stability and compliance while optimizing risk management practices.
Key Takeaways
- Automated financial spreading tools cut processing time and reduce errors in risk assessment.
- AI-driven analysis offers deeper insights and strengthens decision-making for financial institutions.
- Real-time data processing enables swift responses to market changes and supports regulatory compliance, ensuring institutions remain agile and responsive.
Overview of Automating Financial Spreading
Automation in financial spreading reshapes how financial institutions assess and interpret financial statements, allowing analysts to dedicate more time to analyzing trends and making impactful recommendations.
Understanding Financial Spreading
Financial spreading involves extracting data from financial statements and organizing it into a consistent format for analysis. It includes spreading income statements, balance sheets, and cash flow statements to calculate important ratios and metrics critical in financial analysis and forecasting.
Financial analysts rely on this structured data to compare industries and timeframes, forming the basis for credit risk assessments, loan underwriting, and investment decisions. Historically, this has been a manual, time-consuming process with a high potential for errors and discrepancies. The need for financial spreading automation has become even more apparent as data volumes increase and the complexity of financial data grows.
The Need for Financial Spreading Automation
The vast amount of financial data processed by modern institutions demands automation. Manual methods can no longer match the speed and scale needed today. Financial spreading automation addresses key challenges such as:
- Efficiency: Automated systems process financial statements in minutes instead of hours, allowing analysts to focus on strategic tasks.
- Accuracy: AI tools minimize errors in data entry and calculations, resulting in more reliable financial reports.
- Consistency: Standardized processes ensure uniform analysis across financial statements, reducing interpretation discrepancies.
- Real-time insights: Financial spreading automation provides timely updates as new financial data becomes available, allowing quick, well-informed actions.
Automation has significantly cut down processing times and improved analysis quality. Analysts can now focus on interpreting results and strategic initiatives, enhancing commercial lending and risk management decision-making.
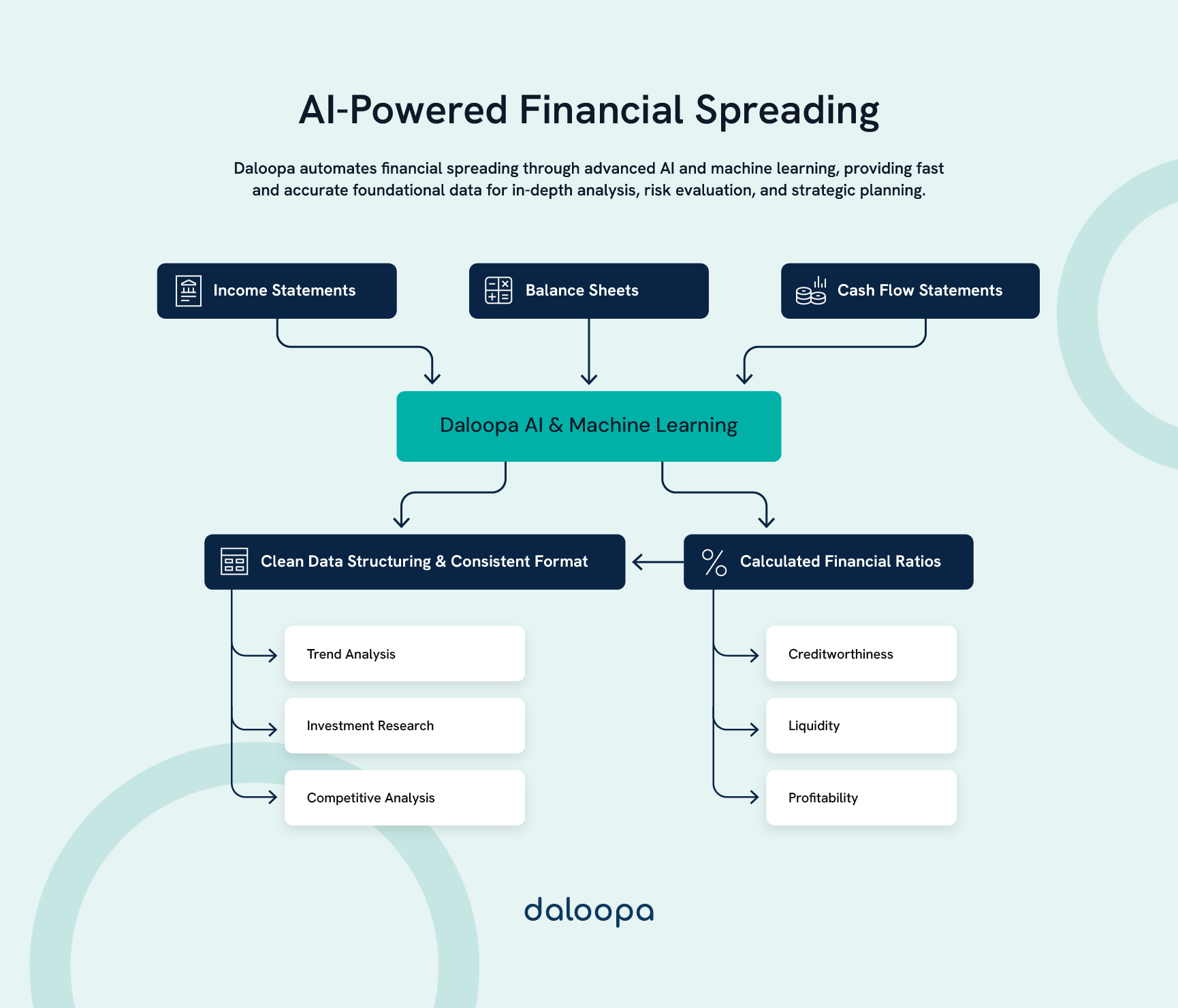
Key Tools and Technologies in Automating Financial Spreading
Automation in financial spreading depends on various advanced technologies that streamline data extraction, processing, and analysis, reshaping risk assessment and decision-making.
AI and Machine Learning in Financial Spreading
AI and machine learning technologies excel at recognizing patterns and interpreting data, boosting the speed and accuracy of analysis. Machine learning models continuously evolve, learning from each financial statement they process, improving precision and relevance with time.
Advanced algorithms process large volumes of data quickly, identifying trends and key metrics that may need to be noticed by human analysts. Machine learning enhances efficiency and enriches the depth of insights that financial professionals can draw from the data they handle. Tools utilize natural language processing (NLP) to extract information from unstructured documents, significantly cutting manual data entry time and minimizing errors.
Cloud-Based Solutions
Cloud-based financial spreading software provides scalability and accessibility, allowing teams to collaborate in real time and maintain consistent data usage across an organization. The shift to cloud solutions also supports remote work, a necessity in the evolving workplace landscape, enhancing productivity and data consistency.
Cloud platforms come with robust security measures, ensuring that sensitive financial data remains protected while allowing authorized users secure access. These solutions integrate easily with other financial tools, supporting seamless workflows and enhanced data management. Regular updates ensure that users benefit from the most current features and compliance standards without the need for disruptive manual upgrades.
Data Visualization Tools
Data visualization tools make complex financial data more understandable. They help analysts quickly identify trends, outliers, and relationships within financial statements, offering a more comprehensive financial health and risks perspective.
Interactive dashboards enable deeper exploration of specific data points, offering a full view of a company’s financial health. Custom charts and graphs help tailor data presentations for various audiences or reporting needs, making communication with stakeholders clearer and more impactful.
Real-time integration ensures visualizations reflect the latest data, supporting timely decision-making in fast-moving financial environments where data relevance is key to strategic moves.
Benefits of Automating Financial Spreading
Automating financial spreading offers significant advantages, transforming how financial institutions perform credit analysis and risk assessments. By using advanced technologies, organizations can achieve greater efficiency, better decision-making, and a higher standard of work quality.
Improved Accuracy and Consistency
Automation significantly reduces mistakes, resulting in more accurate and reliable financial data analysis and better credit assessments and lending decisions, helping institutions manage risks more effectively and maintain credibility.
AI-driven algorithms identify inconsistencies that manual processes may overlook, supporting more reliable risk evaluations. Standardization ensures analysts use uniform methods, avoiding discrepancies in data interpretation. The overall consistency contributes to a clearer understanding of trends and patterns that could influence future financial strategies.
Automation also ensures real-time data updates, enabling credit decisions based on the most current information for a clearer picture of a company’s financial status providing decision-makers with the assurance that evaluations are grounded in the latest financial data.
Enhanced Processing Speed
Automated systems outperform manual methods in processing and analyzing financial data. Benefits include:
- Faster credit decisions and underwriting processes
- The ability to manage larger volumes of financial data, facilitating more comprehensive portfolio management
- Quick identification of high-risk or promising clients, allowing for more strategic allocation of resources
These efficiency improvements allow institutions to respond rapidly to market shifts and customer needs, boosting competitive advantage. Expediting these processes without sacrificing quality allows institutions to maintain a proactive stance in a dynamic financial market.
Compliance and Security Measures
Automated financial spreading tools have built-in compliance checks and strong security protocols, ensuring data processing aligns with regulations and protects client information, maintaining trust and accountability.
Automating compliance reduces the risk of regulatory violations and fines. Automated systems can be quickly updated to meet new regulations, maintaining compliance with minimal manual effort and ensuring that institutions always meet current standards.
Security measures such as encryption, access controls, and audit trails safeguard sensitive data and provide clear records for internal and external audits. These features build client trust and ensure the institution’s integrity during reviews or assessments by regulatory bodies.
Challenges and Considerations
While automating financial spreading offers many benefits, institutions must address certain challenges. Integration and data reliability are key considerations for successful implementation and continued efficiency.
Integration with Existing Systems
Connecting new automated tools with legacy systems can be challenging. Compatibility issues may arise, requiring detailed planning and sometimes custom development to bridge technological gaps and maintain workflow efficiency.
Many institutions still rely on a mix of manual and automated processes. Bridging this gap requires thorough documentation and staff training to ensure smooth transitions and minimize disruptions.
Legacy systems without suitable APIs can create data exchange difficulties, calling for interim solutions or full system upgrades. Planning for these integration challenges helps institutions implement tools smoothly and maximize the value derived from investment in automation.
Data Quality and Source Reliability
The principle “garbage in, garbage out” applies strongly to AI-powered financial spreading. Ensuring high-quality input data is essential for accurate output. Low-quality data inputs can undermine the accuracy and usefulness of automation tools, making source verification crucial.
Financial statements from different sources often come in varied formats, which can lead to parsing errors. Robust data governance practices should be established to minimize these risks.
Multiple data sources bring reliability concerns. Establishing a hierarchy of trusted sources and cross-referencing data can help maintain accuracy. Regular audits and spot checks help ensure that the data fed into automated systems remains clean and reliable over time, supporting continuous, high-quality output.
Manual errors at the source level can still affect automated systems, so regular audits and spot checks are necessary to identify and correct issues before they propagate, maintaining the credibility of analyses.
Case Studies and Real-World Applications
Successful implementations of AI-powered financial spreading tools provide insights for institutions considering similar transitions. These examples illustrate the potential benefits and the strategic approaches that can be adopted for smooth execution.
Successful Implementations
JPMorgan Chase – COiN (Contract Intelligence) Platform
JPMorgan Chase developed COiN, an AI-driven platform, to streamline and automate data extraction from financial documents, particularly in contract review for loan arrangements. Using machine learning, COiN processes thousands of complex contracts in seconds, which would have manually taken legal teams up to 360,000 hours, allowing JPMorgan to reassign resources to more value-adding activities, cut operational costs, and improve data accuracy.
ING – Use of Machine Learning in Risk Modeling
ING Bank adopted machine learning for its credit risk modeling processes, transforming how it forecasts loan default risk. This automation has reduced the time required for model updates and validation, enabling more agile responses to regulatory changes and improving the accuracy of credit risk assessments. ING’s use of machine learning has positioned the bank to improve operational efficiency and comply more effectively with evolving regulatory demands.
Lessons Learned
Training and User Adoption: As demonstrated by JPMorgan, comprehensive training and clear onboarding for end-users proved essential. Both institutions reported that early user involvement and targeted training programs helped mitigate user adoption challenges and increased ROI.
Data Readiness: ING’s experience with machine learning highlighted the importance of high-quality, standardized data for accurate and reliable output. ING ensured that its data processes were streamlined and that the AI models were trained on clean, consistent datasets, which improved model reliability.
These real-life examples underscore the tangible benefits of automation and AI-powered financial spreading, from improved operational speed to greater data accuracy. As financial institutions continue to adopt these tools, they can reduce manual tasks, ensure regulatory compliance, and enhance competitive edge through faster, more reliable financial analyses.
The Impact of Automation
Automation is revolutionizing financial spreading, enhancing efficiency and accuracy in risk assessment processes. It reshapes how financial institutions handle data, make strategic decisions, and maintain compliance, fostering a forward-thinking approach to data analysis.
Adopt Automation Tools for Your Fundamental Data Needs
Adopting automation tools for financial spreading extract data from various financial documents, standardize it, and populate spreadsheets with minimal human effort, optimizing the workflow.
Accuracy improves significantly with automation. Machine learning algorithms can accurately identify and categorize financial data, reducing errors typical of manual processes, leading to more reliable risk assessments and better-informed lending decisions, and contributing to sounder financial operations.
Automation enables real-time data processing. Financial statements can be analyzed immediately, allowing for rapid responses to market changes and emerging risks. This speed is crucial in today’s dynamic financial environment, where timely decision-making can be a major differentiator.
Compliance management also benefits from automation. These tools can be programmed to follow specific regulatory requirements, ensuring consistency in reporting and reducing the risk of non-compliance. This feature is especially valuable as financial regulations evolve, helping institutions stay ahead in the regulatory landscape while fostering a culture of trust and transparency.
Automating Financial Spreading with Daloopa
Imagine reducing your financial analysis time from hours to minutes. Daloopa leverages advanced machine learning, cloud accessibility, and sophisticated data visualization to automate financial spreading, making it faster, more reliable, and highly adaptable. Daloopa’s automation tools streamline workflows by extracting and structuring data from various document types, minimizing manual input, and reducing errors associated with traditional methods.
With real-time insights and dynamic data updates, Daloopa empowers financial professionals to stay ahead in a fast-paced environment, allowing more time for strategic analysis and decision-making. Transform financial analysis into a seamless, efficient process with Daloopa’s powerful automation capabilities.
Learn how Daloopa’s advanced tools for automating financial spreading can streamline your workflow, minimize errors, and automate financial spreading to keep you ahead in a fast-paced financial landscape.