Data analytics is now a fundamental aspect of modern banking and financial services. With intensifying competition and changing customer expectations, the strategic application of big data has become a considerable advantage. Through advanced analytics, banks and financial firms can discover insights that guide smarter decisions, bolster risk management, and deliver tailored customer experiences.
Financial institutions are moving beyond simple reporting toward using advanced analytics tools to predict trends, seize opportunities, and proactively manage risks. For instance, a McKinsey pilot test found that some banks grew their portfolio by 9% in a year and reduced account planning time by 90% with data and advanced analytics. This shift is powered by predictive analytics in finance, enabling institutions to forecast market movements and make data-driven decisions
Integrating data analytics into key banking operations is transforming the industry. From fraud detection to developing new products, data-driven strategies empower financial services to function with enhanced precision and efficiency. As the digital landscape continues to grow, institutions that master data analytics will lead in adapting to customer demands and operational innovations.
Key Takeaways
- Data analytics supports financial institutions in making data-driven decisions and delivering personalized services.
- Advanced analytics tools are essential for forecasting trends and managing risks in banking.
- Proficiency in data analytics is critical for financial institutions to remain competitive in the digital age.
How Data Analytics in Banking Drives Efficiency and Innovation
Data analytics is now foundational in banking and financial services, enabling institutions to make well-informed decisions, improve risk management, and design customized customer experiences.
Enhancing Risk Assessment and Mitigation
Risk management has transformed with data analytics. Financial institutions can analyze vast sets of information, identify potential risks more accurately, and craft effective mitigation strategies.
Advanced tools enhance credit risk assessments by analyzing borrowers’ financial records, transaction patterns, and economic indicators. This leads to more precise lending decisions and reduces the likelihood of defaults.
Real-time analytics monitor market trends and spot anomalies that could signal financial risks. For example, HSBC uses AI to monitor and analyze billions of transactions monthly to flag unusual transaction patterns and detect crime with improved accuracy. As a result, they are now discovering about three times more crime with 60% fewer false positives.
This capability not only protects against sudden losses but also increases customer trust through proactive fraud prevention.
Big data also strengthens stress testing models, helping institutions prepare for different economic scenarios. Improved models ensure institutions can comply with regulatory requirements while maintaining a solid approach to financial risk.
Fraud Detection
The effectiveness of fraud detection has significantly increased with data analytics. Sophisticated algorithms now evaluate transaction patterns to identify suspicious activities. J.P. Morgan, for example, uses synthetic data and AI-driven analytics to identify fraud patterns in payment transactions. By simulating various fraud scenarios, they detect questionable behaviors in real time, protecting both the bank and its customers.
Machine learning models reveal subtle anomalies that could indicate fraud, such as unusual spending habits or attempts to access accounts from unfamiliar locations. This ability to detect irregularities early minimizes financial losses and builds customer trust.
Analyzing historical fraud data helps institutions develop predictive models that anticipate new fraud methods. These models continually evolve, adapting to emerging fraud tactics.
Real-time analytics block potentially fraudulent transactions instantly, preventing unauthorized access and minimizing potential losses, which keeps customer assets secure.
Personalizing Customer Experiences
Banks use data analytics to offer highly customized customer experiences. They can uncover insights into customer preferences and needs by examining transaction histories, browsing habits, and other data points.
Banks use these insights to make relevant product recommendations, offer personalized financial advice, and execute targeted marketing campaigns, which boost customer satisfaction and loyalty.
Advanced segmentation divides customers into smaller, more specific groups, allowing banks to create precise, personalized offerings. This leads to more meaningful interactions that resonate with individual customer needs.
Predictive analytics in finance enables banks to anticipate future customer needs, making proactive recommendations for products or services. This approach strengthens opportunities for cross-selling and upselling, benefiting both customers and the institution.
Optimizing Operational Efficiency Through Data Analytics in Banking
Data analytics improves operational efficiency across the organization. Financial institutions apply advanced tools to optimize resource allocation, streamline internal processes, and automate repetitive tasks, reducing operational costs.
By analyzing customer service data, banks can identify recurring issues and improve support processes, reducing wait times and enhancing the customer experience. For instance, Citigroup transformed client service with data analytics, using digital platforms to streamline service requests and address client needs more quickly. Through a combination of data analysis and automation, Citigroup has reduced response times and achieved smoother operations.
Predictive maintenance models also allow banks to foresee equipment failures and schedule preventive maintenance, cutting down on unexpected downtimes and associated costs. According to Deloitte, predictive maintenance can reduce facility downtime by 5-15% and increase labor productivity by 5-20%. Predictive analytics in finance further optimizes operational efficiency by forecasting potential disruptions and streamlining resource allocation.
Additionally, data-driven insights inform decisions about branch locations, ATM placements, and digital service offerings, ensuring that resources align with customer usage patterns and preferences, thereby maximizing service accessibility.
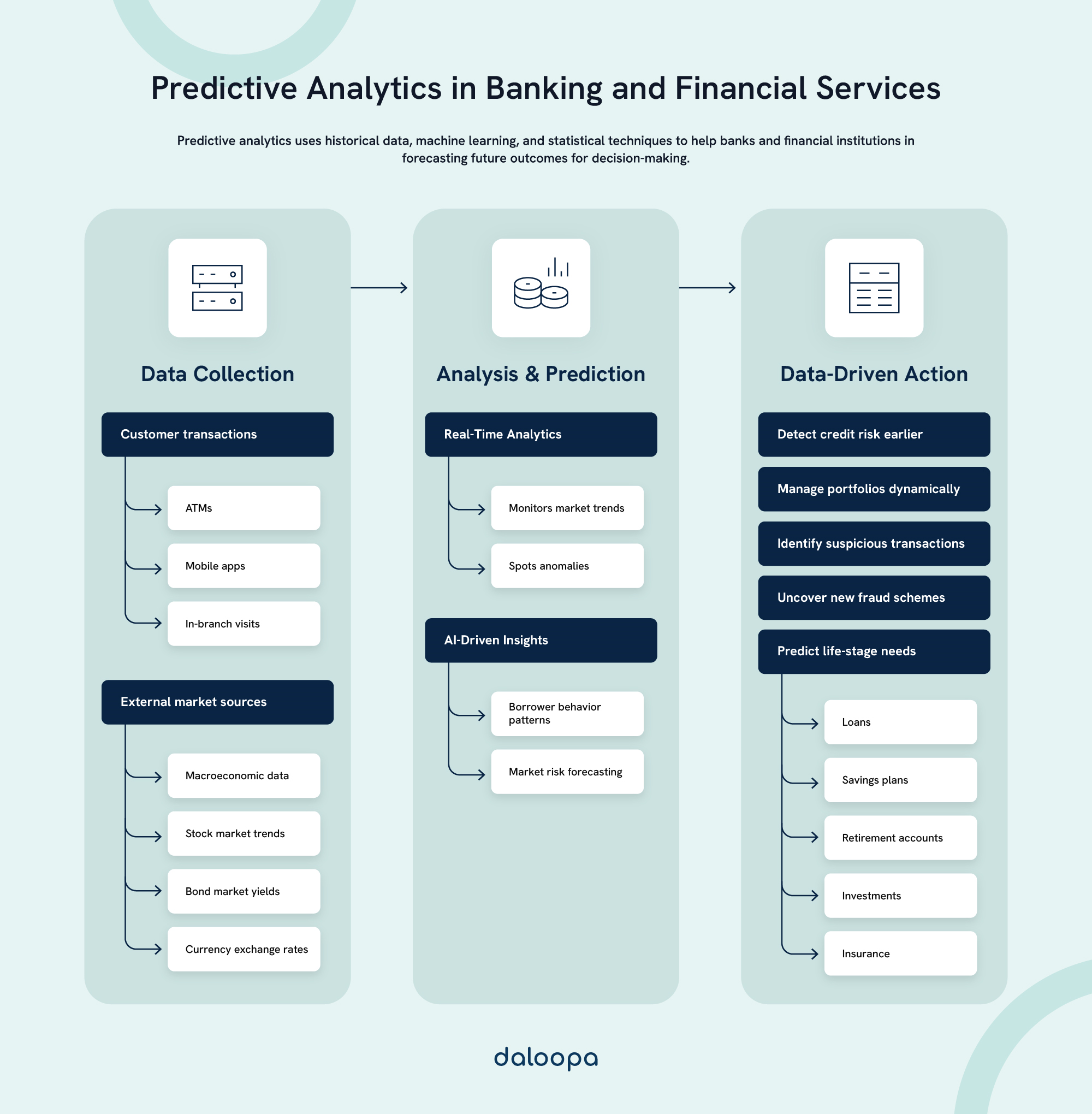
Challenges to Implementing Data Analytics in Banking and Financial Services
Despite its benefits, implementing data analytics in financial institutions presents challenges.
Data quality and management issues: Many financial institutions still rely on older, legacy systems where data is often stored in outdated formats or spread across multiple sources. This can lead to inconsistent or incomplete information, making it difficult to consolidate data for accurate analysis. To address this, institutions can invest in data-cleaning tools and establish standardized data governance practices to ensure data consistency and reliability across all systems.
Regulatory compliance complexity: Businesses collecting and processing personal data must comply with strict data privacy laws like GDPR in Europe and CCPA in the U.S. Full disclosure and customer consent is required for data use. In addition to strong data governance policies, financial institutions need to use secure data storage and encryption technologies. They should also perform regular audits and compliance training to help teams stay up-to-date with evolving regulations and avoid costly penalties.
Cultural resistance to change: Shifting to data-centric operations often requires significant changes in workplace culture, which can be challenging for employees used to traditional processes. Financial institutions can overcome this resistance by providing ongoing training on data analytics and emphasizing the benefits of data-driven insights. Leadership can also foster a culture of innovation, encouraging teams to adopt new tools and approaches as part of a shared goal.
Technical integration challenges: Integrating advanced analytics into existing systems can be costly and complex, especially for older data architectures not designed for modern analytics. To simplify the process, institutions can adopt cloud-based analytics platforms that are easier to scale and integrate with legacy systems. Partnering with technology providers or consulting firms can also provide the expertise to navigate complex integrations.
Talent acquisition and retention: The demand for skilled data scientists and analysts in finance continues to grow, outpacing supply. This can make it difficult to recruit and retain talent. Financial institutions can address this by investing in employee development programs and fostering partnerships with universities to tap into emerging talent pools. Offering competitive compensation and creating a collaborative, growth-oriented work environment can also help retain top talent.
Data privacy and skilled concerns: With vast amounts of sensitive information being collected and analyzed, data breaches are a significant risk. Strong cybersecurity measures, including data encryption, multi-factor authentication, and regular security audits, are essential to protect customer information. Institutions can also use anonymization and tokenization to safeguard data during analysis without compromising privacy.
Strategies for Staying Competitive in a Digital Landscape
Banks must embrace advanced technologies and data-driven practices to maintain a competitive edge. Key strategies focus on big data, AI, and creating a strong data ecosystem to drive innovation.
Developing Data-Driven Strategies
Data-driven strategies are essential for banks to make informed decisions and stay ahead. Collecting and analyzing customer data reveals actionable insights that help personalize services, improve risk management, and pinpoint new market opportunities.
Real-time analysis supports swift responses to changes in the market and customer needs, enabling optimized pricing and strengthened fraud prevention capabilities.
Fostering a data-centric culture within the organization requires staff training in data literacy and promoting data-driven decision-making across departments.
Using Advanced AI and Machine Learning
Artificial intelligence and machine learning are revolutionizing banking. These technologies enable process automation, enrich customer experiences, and uncover valuable insights from large data volumes.
AI-powered chatbots offer round-the-clock customer service and personalized recommendations. Machine learning models improve credit scoring, detect transaction anomalies, and enhance investment strategies.
To maximize AI’s benefits, banks should:
- Invest in AI and machine learning expertise.
- Align AI applications with core business goals.
- Build a robust data governance framework.
- Monitor and refine AI models continuously.
Building an Efficient and Robust Data Ecosystem
An efficient data ecosystem is vital for successful analytics and AI initiatives. Building a centralized platform that integrates data from across the organization is essential. For instance, a well-integrated data ecosystem can combine insights from customer interactions across ATMs, apps, and branches, creating a comprehensive view that supports personalized banking experiences.
Key elements of a robust data ecosystem include:
- Data lakes for diverse data storage.
- Advanced processing tools for real-time analysis.
- Secure data sharing for collaboration.
- Rigorous data quality management systems.
A Chief Data Officer can steer data initiatives and ensure alignment with business strategies. Data engineers and scientists play a crucial role in maintaining this ecosystem and advancing data-driven goals.
The Future of Data Analytics in Banking
The future of banking is unmistakably data-driven. Data analytics is reshaping banking and financial services by driving smarter decisions, personalizing customer experiences, and enhancing risk management. With advanced tools, financial institutions can now predict trends, detect fraud, and streamline operations, creating a stronger and more responsive sector. Although challenges like regulatory compliance and technical integration persist, strategies such as adopting AI and building robust data ecosystems are helping banks navigate these obstacles.
As data analytics in banking and financial services continues to evolve, it remains a crucial asset for institutions aiming to stay competitive and meet modern customer demands.
To achieve this, professionals need reliable and comprehensive data to inform their analyses. Daloopa’s AI-driven data analytics platform empowers banking and finance teams by providing fast, up-to-date, and complete data for quicker, smarter decision-making. With Daloopa’s support, you can unlock the full potential of data analytics to drive sustainable growth and innovation.
Learn how our solutions enhance data analytics in banking and finance. Book a demo today!