What if one erroneous data point costs your institution millions in regulatory fines or, even worse, erodes your client trust overnight? Financial organizations cannot afford to take data quality for granted in an industry where precision is paramount. Accurate, timely, and consistent data forms the backbone of every critical decision—from managing risk and ensuring compliance to maintaining client confidence and protecting your reputation.
Financial services leaders understand that data quality engineering in financial services is not a luxury but a necessity. By adopting robust data quality engineering practices, institutions safeguard their operations and create a competitive edge in today’s data-driven environment.
Key Takeaways
- Reliable data drives smarter decision-making: Accurate data minimizes errors and strengthens compliance, ensuring every financial decision is built on a trustworthy foundation.
- A structured approach prevents costly mistakes: Clear data quality specifications, automated quality controls, and ongoing monitoring help avoid regulatory violations, trading errors, and reputational damage.
- Company-wide buy-in and advanced tools are essential: Empowering every team member—from data engineers to top executives—with proper training and cutting-edge technologies sets the stage for sustainable improvements.
The Basics of Data Quality
Data quality forms the cornerstone of successful financial operations. Data quality engineering in financial services ensures accuracy, consistency, and compliance, reducing risks and enhancing decision-making. It rests on four key dimensions:
- Accuracy: Data must be correct and precise.
- Completeness: All necessary data should be present.
- Consistency: Data across systems must align with defined standards.
- Timeliness: Data must be available when needed for decision-making.
Focusing on these dimensions lays a strong foundation for dependable operations and sustainable growth. For instance, a well-structured data quality framework prevents an analyst from using outdated figures that could lead to an incorrect risk assessment.
Challenges in Financial Data Management
Financial institutions encounter a myriad of challenges when managing data:
Incomplete or Missing Information
Incomplete data can distort risk assessments. Imagine a scenario where a crucial transaction record is missing—this gap could lead to underestimating exposure to risky assets, potentially resulting in significant financial losses.
Inconsistencies Between Systems
Different systems often store data in incompatible formats. These inconsistencies lead to reporting errors and misinterpretations. For example, when a bank merges with another institution, legacy systems may use different definitions for the same metrics, making it difficult to consolidate data reliably.
Outdated Data and Compliance Risks
Not updating data regularly can violate regulations such as GDPR or BCBS 239. Outdated data jeopardizes compliance and can trigger severe regulatory fines, not to mention reputational damage. A single instance of non-compliance could tarnish a financial institution’s reputation for reliability.
Persistent Data Silos
Data silos—where information is isolated in separate systems—prevent a unified view of client relationships and exposures. Integrating legacy systems becomes even more challenging during mergers and acquisitions, increasing the risk of inconsistent data that undermines decision-making.
Diverse Data Sources
Financial data is not homogenous. It includes structured records (like transactions) and unstructured sources (such as client emails). Managing this diversity requires advanced tools and quality control processes to integrate disparate data types into a cohesive dataset.
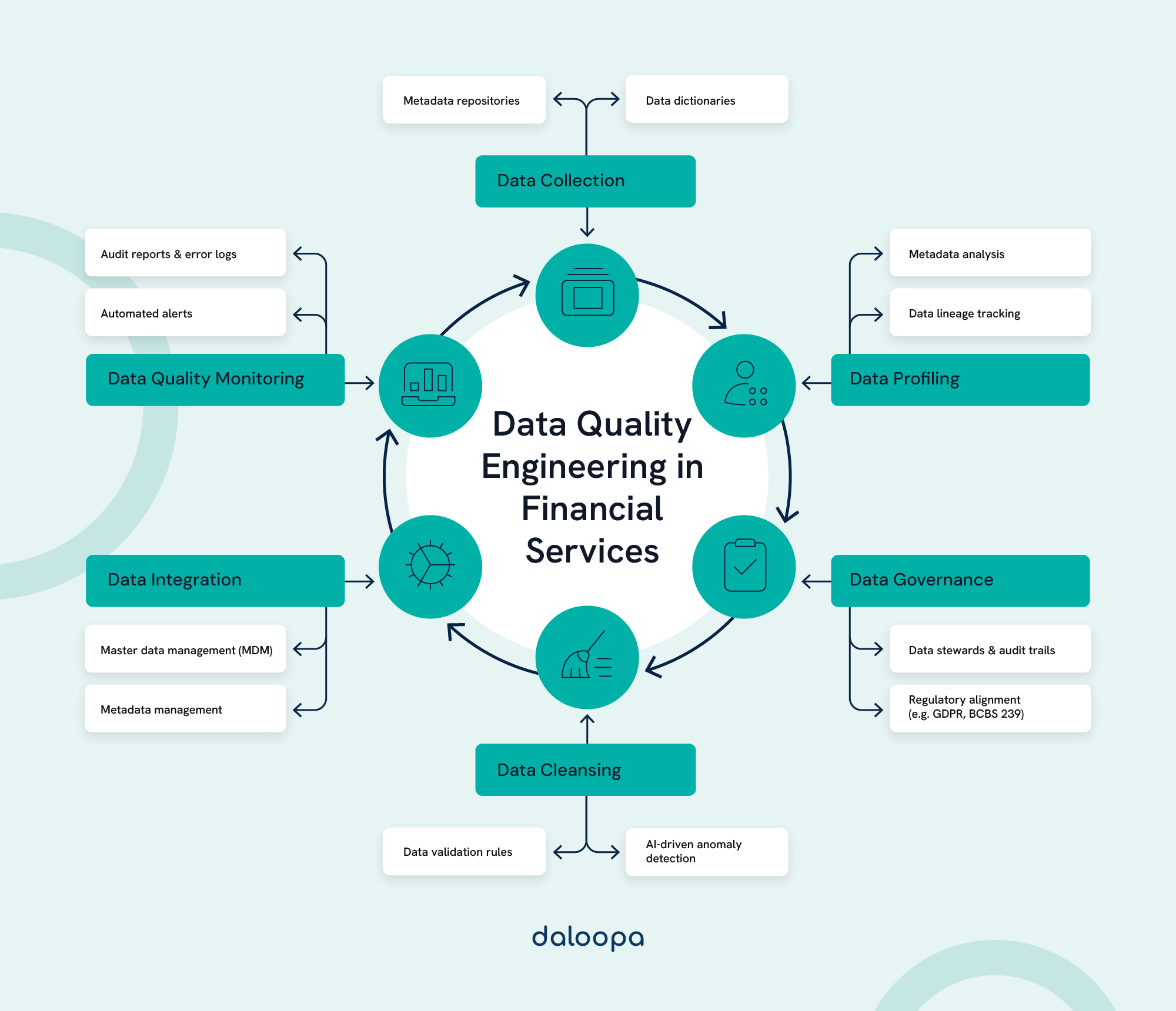
Frameworks and Tools for Data Quality Engineering in Financial Services
Financial institutions must rely on effective frameworks and tools to ensure data accuracy and reliability. A comprehensive approach ensures data remains valuable across all functions, from risk management to client servicing.
Financial Data Quality Specifications
Data Quality Specifications (DQS) are the blueprint for establishing clear quality standards. They include:
- Metadata Definitions: Clearly defined descriptions for every data field.
- Acceptable Value Ranges: Limits within which data values must fall.
- Data Format Guidelines: Standardized formats to ensure consistency.
- Consistency Rules: Cross-dataset rules that guarantee uniform interpretation.
These specifications set clear expectations for data engineers, analysts, and stakeholders. By using centralized data catalogs to manage metadata, institutions can easily update standards as business needs evolve. Moreover, well-documented DQS simplify compliance reporting, as regulators increasingly require detailed explanations of data sources and validation processes. This proactive approach meets regulatory demands and boosts overall operational efficiency.
Data Profiling and Quality Checks
Data profiling tools analyze the structure, content, and quality of datasets. They help organizations:
- Identify Missing or Incomplete Data: Detecting gaps before they affect analysis.
- Detect Anomalies and Outliers: For example, a hypothetical case might involve a machine learning algorithm identifying an unusually high number of transactions from a particular branch—this could signal fraud.
- Assess Data Patterns and Distributions: Recognizing trends that inform business decisions.
- Validate Data Against Established Specifications: Ensuring every incoming data point meets pre-defined standards.
Automating quality checks and integrating them into processing pipelines means that data is verified in real-time before it informs critical decisions. Real-time monitoring solutions further enhance reliability by alerting teams to emerging issues before they lead to significant disruptions.
Best Practices for Ensuring Financial Data Quality
To uphold high-quality data standards, financial institutions should adopt these proven strategies:
Implementing Robust Data Governance Policies
Data governance is essential for achieving lasting data quality. Organizations must:
- Assign Clear Roles and Responsibilities: Define who owns each part of the data lifecycle.
- Establish a Comprehensive DQS Document: This document details quality tolerances, dimensions, and validation rules everyone must follow.
- Track Data Lineage: Knowing the origin and evolution of data enables teams to pinpoint and correct issues at the source.
- Promote Consistent Definitions and Formats: Uniform standards across the organization reduce misunderstandings and ensure that every department speaks the same “data language.”
Strong data governance enhances audit readiness and bolsters compliance, a critical advantage in highly regulated financial markets.
Regular Data Monitoring and Auditing
Continuous monitoring helps organizations maintain quality over time. Automated tools can assess metrics like completeness and consistency in real-time, flagging anomalies as they occur. Periodic audits, meanwhile, provide deeper insights into systemic issues and areas for improvement.
Scorecards tracking financial data quality indicators are valuable for measuring progress and identifying trends. Establishing a feedback loop with users helps prioritize quality concerns and refine processes. Institutions can build a more resilient data infrastructure by addressing these concerns collaboratively.
Integrating quality checkpoints throughout data workflows ensures errors are addressed early, preventing downstream issues. These checkpoints can be customized to address specific risks, providing a tailored approach to maintaining quality.
Leveraging Modern Financial Data Management Techniques
Modern technologies significantly enhance data quality efforts. Data quality engineering in financial services leverages advanced financial data management tools such as AI-driven anomaly detection, automated data cleansing, and real-time validation systems. For example:
- Machine Learning Algorithms: These can automatically detect patterns and anomalies in vast datasets.
- Master Data Management (MDM) Systems: MDM establishes a single source of truth for key business entities, reducing data inconsistencies.
- Data Quality Rules Engines: These automate validation processes, ensuring that quality standards are applied uniformly across all data.
- Metadata Management Tools: Such tools improve transparency, enabling authorized users to locate and trust the data they need quickly.
- Advanced Analytics Platforms: These extract actionable insights from high-quality data, transforming quality improvements into competitive advantages.
The combination of these modern financial data management techniques supports compliance and risk management and positions financial institutions to harness data as a strategic asset.
Impact of Data Quality on Financial Services
Data quality has a direct and significant impact on the financial services sector. It influences every aspect of operations, from internal workflows to external client interactions.
Poor data quality can lead to errors that disrupt decision-making, increase compliance risks, and damage an organization’s reputation. In contrast, high-quality data enhances operational efficiency, strengthens regulatory compliance, and builds the trust necessary to attract and retain clients.
By prioritizing data quality engineering in financial services, organizations can implement robust data governance frameworks, automate validation processes, and leverage advanced analytics to ensure data integrity. This proactive approach minimizes financial risks and improves overall business performance.
Empowering Accurate Decision-Making
Reliable data empowers institutions to make confident, informed decisions. Clean, consistent datasets lead to better risk management, more effective investment strategies, and superior client service outcomes. For example:
- Risk Management: Accurate data ensures that risk assessments reflect true exposures, preventing miscalculations that could result in financial losses.
- Investment Strategies: Consistent data supports more reliable forecasts, enabling institutions to identify profitable opportunities and avoid costly errors.
- Client Trust: High-quality data reduces the need for manual corrections, thereby preserving the institution’s reputation and strengthening client relationships.
By prioritizing data quality engineering in financial services, financial organizations reduce the likelihood of trading errors or regulatory missteps, ultimately lowering operational costs and enhancing their competitive edge.
How Daloopa Can Help Transform Your Data Quality
Transform your financial data processes today. Visit Daloopa to learn how our AI-powered platform can automate your fundamental data updates, streamline your model-building process, and give you the complete, accurate, and fast data you need to stay ahead in the competitive world of financial services. Request a demo now, and let Daloopa be your co-pilot for smarter, faster, and more reliable financial modeling.