What if your financial models could predict the future with pinpoint accuracy? For CFOs, analysts, and FP&A teams, artificial intelligence or AI-driven financial tools is turning this once-distant vision into reality. Financial professionals can streamline processes, minimize errors, and extract deeper insights from data by shifting from static, spreadsheet-based models to dynamic AI-powered systems.
Machine learning algorithms and big data analytics enable organizations to process vast financial datasets in real time, uncovering trends and risks that traditional methods often miss. With AI for financial modeling, finance teams can enhance forecasting, optimize portfolio strategies, and improve decision-making at every level.
Yet, AI adoption comes with challenges. Data quality, implementation strategies, and integration with existing systems must be carefully managed. However, the benefits far outweigh the obstacles. By embracing AI, companies future-proof their financial modeling capabilities, ensuring resilience and agility in an evolving market landscape.
Key Takeaways
- AI-driven models improve forecasting and real-time scenario analysis.
- Addressing data quality and implementation strategies is key to successful AI adoption.
- AI empowers teams to focus on strategic priorities by automating routine tasks.
The Shift From Traditional To AI-Driven Models
Traditional methods, while reliable in the past, often struggle to meet the demands of modern financial markets. AI-driven models are stepping up to bridge this gap.
AI automates repetitive tasks, freeing time for strategic analysis. This evolution is more than just about efficiency—it changes how we analyze and predict financial trends.
How Traditional Models Fall Short:
- Relies heavily on historical data, limiting predictive capabilities.
- Requires extensive manual data input, increasing the risk of errors.
- Struggles to incorporate real-time or unstructured data.
How AI-Driven Models Outperform:
- Leverage machine learning to analyze vast datasets and detect trends.
- Automate data updates, reducing manual work and increasing efficiency.
- Adapt dynamically to new market conditions, refining forecasts in real-time.
For example, a multinational consumer goods company (hypothetical scenario) using traditional financial models may take days to update forecasts after a major supply chain disruption. In contrast, an AI-driven model could instantly process real-time logistics data, predict the financial impact, and suggest mitigation strategies—giving decision-makers a crucial head start.
Hybrid approaches are also emerging, blending human expertise with AI-powered insights to maximize AI’s computational strengths while retaining the strategic oversight of finance professionals.
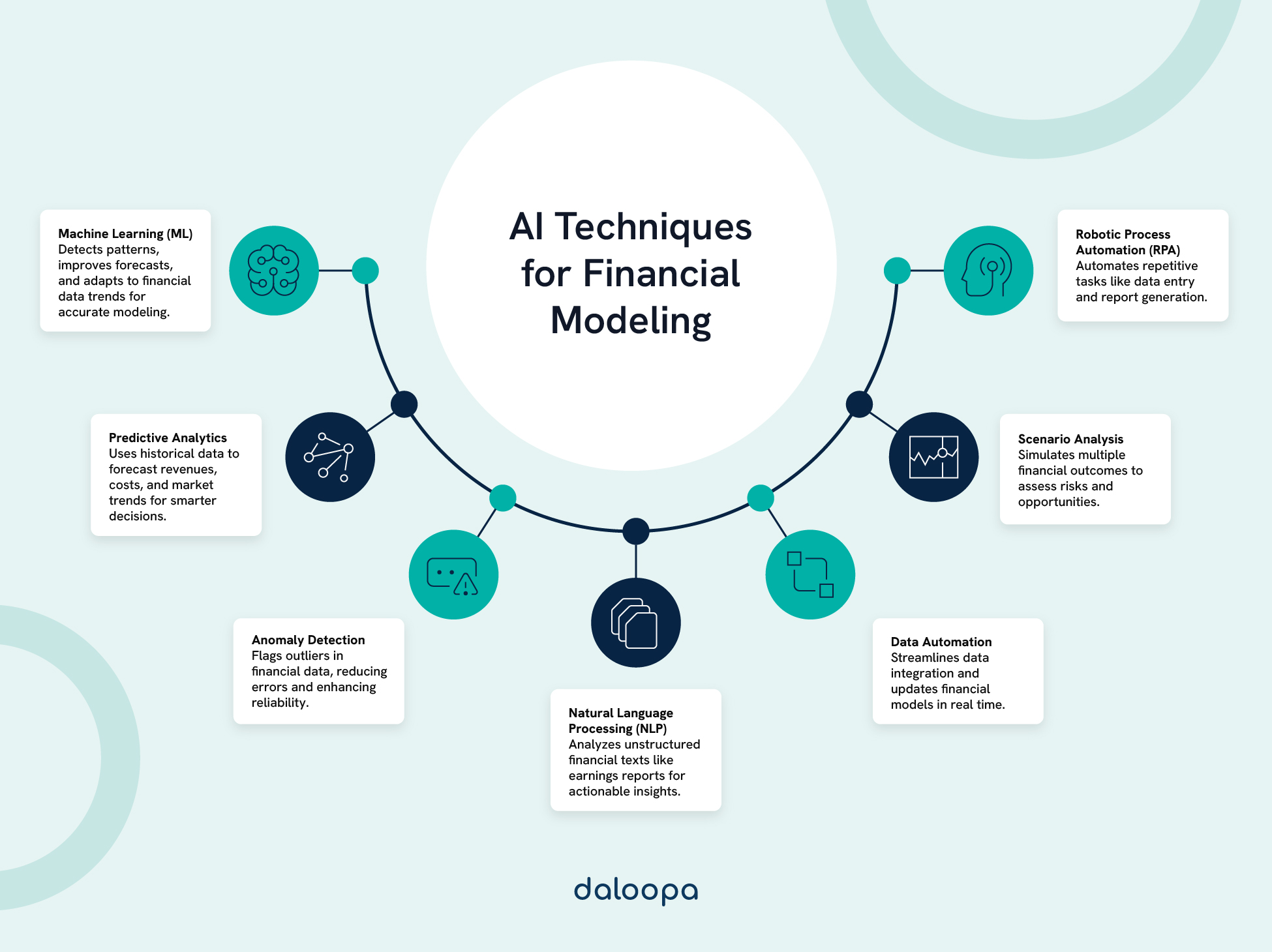
Financial Modeling Techniques with AI
Financial modeling techniques with AI have revolutionized traditional financial modeling. These advancements bring increased accuracy, efficiency, and actionable insights.
Automating Repetitive Tasks
AI for financial modeling dramatically improves operational workflows by handling time-consuming tasks such as:
- Automating data collection and cleansing, ensuring consistency and accuracy.
- Generating reports with standardized formatting, reducing human error.
- Updating financial models dynamically, reflecting real-time market shifts.
By eliminating manual bottlenecks, AI allows analysts to focus on strategic planning rather than repetitive administrative work.
Enhancing Forecasting Accuracy
AI significantly improves forecasting precision. Machine learning and deep learning algorithms uncover patterns in historical data that traditional methods might miss.
Key advantages include:
- More accurate revenue and expense predictions
- Improved cash flow projections
- Enhanced earnings estimates
These forecasts incorporate broader variables and adapt to market changes faster, enabling smarter resource allocation and investment decisions. For instance, AI models can simultaneously account for macroeconomic indicators, competitive activity, and customer behavior trends, offering a richer, multidimensional forecast.
Real-Time Scenario Analysis
AI allows finance teams to model multiple scenarios instantly, enhancing agility in decision-making. Organizations use AI to:
- Assess the impact of economic changes (e.g., inflation, interest rate hikes).
- Evaluate potential investments and their financial repercussions.
- Analyze regulatory policy changes in real-time.
The ability to quickly adapt and make data-driven decisions equips companies to navigate volatile markets effectively. For instance, a business can analyze how sudden shifts in interest rates or supply chain disruptions might impact profitability, enabling them to mitigate risks proactively.
Improving Risk Management
Financial modeling techniques with AI enhance risk management frameworks by introducing sophisticated AI-driven financial tools for accurate assessment and mitigation.
Enhancements include:
- Advanced credit risk modeling
- Effective fraud detection systems
- Comprehensive stress-testing methodologies
AI’s ability to process extensive datasets uncovers risks that might remain hidden, enabling more resilient financial strategies. For example, AI models can identify anomalies in transaction data that suggest potential fraud, allowing organizations to intervene before damage occurs.
Tools And Technologies Empowering AI In Financial Modeling
AI innovations transform financial modeling by offering unprecedented analytical capabilities, dynamic forecasting, and streamlined decision-making.
AI-Powered Platforms And Software
AI-driven platforms are reshaping financial modeling tools. While Excel remains a staple, its integration with AI capabilities elevates its functionality. AI-enhanced software solutions, like Daloopa, enhance financial projections and model validation.
Key features include:
- Big data processing
- Advanced visualization tools, often integrated with Power BI
- Natural language processing for analyzing unstructured data (e.g., news or reports)
These technologies allow finance professionals to gather deeper insights and present them intuitively. Additionally, AI-powered platforms are increasingly equipped with customizable dashboards, enabling users to monitor key performance indicators and track trends in real time.
Integration Of AI With Existing Systems
Integrating AI with legacy financial systems ensures a seamless shift to enhanced modeling processes. Tailored AI solutions complement traditional tools, enabling organizations to achieve more without replacing established workflows.
Implementation strategies include:
- Customizing AI models to fit specific business needs
- Ensuring compatibility with existing tools
- Training staff to interpret AI-generated insights
Companies can leverage AI without disrupting their operations by bridging traditional and modern approaches. This integration often extends to cloud-based systems, enabling greater collaboration and scalability across departments.
Challenges And Considerations In AI Adoption
AI adoption in financial modeling introduces complexities organizations must address to maximize benefits.
Data Integrity And Quality
Accurate financial models depend on high-quality data. Poor data can skew predictions and impair decision-making.
Best practices include:
- Implementing robust data validation processes
- Conducting regular data pipeline audits
- Automating real-time quality checks
Maintaining reliable datasets is critical, especially for risk-sensitive tasks like credit scoring or fraud detection. Organizations must also ensure that their data is free from biases, as this can directly impact the fairness and reliability of AI predictions.
Implementation Strategies
Effective AI adoption requires a clear strategy. Identifying high-value use cases, such as the video example below, and fostering collaboration between IT and finance teams are essential steps.
Key approaches:
- Starting with pilot projects to test AI-driven financial tools
- Training analysts to work with AI systems
- Refining AI solutions for scalability and adaptability
Strategic implementation ensures AI-driven financial tools align with business objectives and deliver meaningful insights. Additionally, establishing cross-functional AI task forces can accelerate adoption by fostering knowledge-sharing and aligning technical expertise with organizational goals.
Utilizing AI For A Competitive Edge
AI empowers finance professionals with actionable insights, faster decision-making, and enhanced forecasting accuracy. Companies leveraging AI for financial modeling gain a distinct advantage in market analysis, portfolio optimization, and strategic planning.
Predictive analytics enables organizations to anticipate market shifts, simulate potential outcomes, and optimize investment strategies with unprecedented precision. By automating routine tasks, AI frees finance teams to focus on high-value activities that drive business growth.
Furthermore, AI-driven financial tools like Daloopa streamline financial modeling by automating data extraction and updates, ensuring accuracy while reducing manual effort. With AI-driven solutions, finance teams can operate more efficiently, minimizing errors and maximizing insight generation.
Future-Proof Your Financial Models with AI
The future of financial modeling is AI-powered. Organizations that adopt AI-driven solutions position themselves for sustained success in an increasingly data-driven world. Whether optimizing cash flow projections, refining investment strategies, or mitigating risks, AI for financial modeling transforms financial decision-making at every level.
Ready to elevate your financial modeling capabilities? Explore how Daloopa can automate, optimize, and enhance your models for smarter, faster, and more accurate financial forecasting. Visit Daloopa today to learn more.